It's THE word of the moment: AI. On every software publisher's lips and beyond, artificial intelligence is now making its way into the world of Atlassian tools, more specifically within Jira and Confluence. Called Atlassian Intelligence (the initials stand for AI, got it?), it's a set of AI-based functionalities. Atlassian Intelligence is only available on the Cloud version of these tools.Atlassian AI promises to "help companies and teams unlock their full potential". How? By generating content or automating certain repetitive tasks. With its AI integration, Atlassian promises to achieve the impossible. Nothing less.
But what is it really? What features lie behind this ambitious promise?
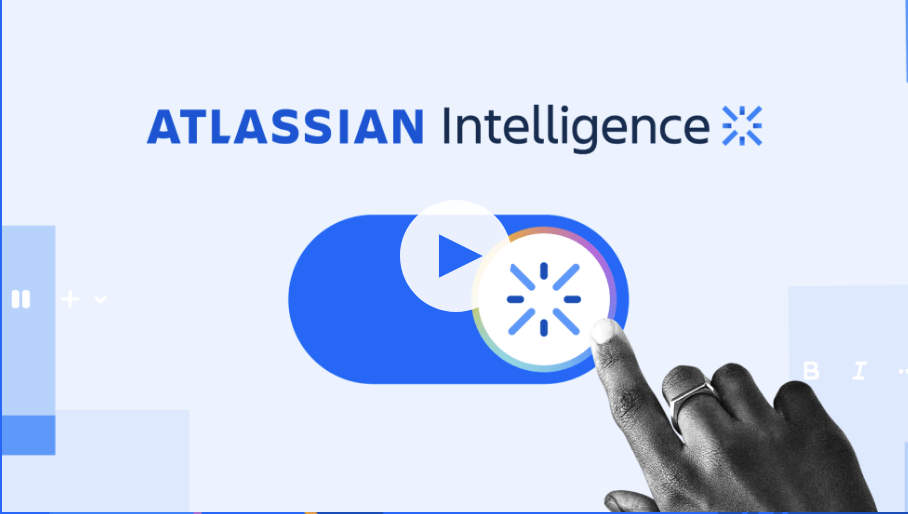
Atlassian turns to artificial intelligence
Announced with great fanfare last April, Atlassian Intelligence has been evolving ever since, just like the OpenAI and other models. So much so, in fact, that it now includes a large number of AI-powered functionalities.
From content generation to the automatic creation of automation rules, to natural language ticket searches, it's easy to get lost and not know how to make the most of these new possibilities. Let's take a look at the new features hidden behind Atlassian Intelligence, Atlassian's new addition for Cloud tools (Premium and Enterprise plans only).
And here's where it gets tricky: Atlassian Intelligence is not available as a Data Center product. It's a decision that seems final. Fortunately, this doesn't mean you have to say goodbye to AI. Users of the Data Center version can still take advantage of other solutions to integrate AI into their processes. With this in mind, it is important to emphasize that most of the functionalities offered by Atlassian Intelligence are based on linguistic models developed by OpenAI. They enable natural language to be analyzed and generated in Atlassian's Cloud products.
Atlassian and the data protection policy
Before getting to the heart of the matter, what is Atlassian's policy on the protection of user data concerning Atlassian Intelligence?
An important question to ask, given that data protection and ethics in AI is still a thorny issue. As we have seen, Atlassian Intelligence relies on OpenAI's linguistic models. This collaboration enables Atlassian Intelligence to deduce interactions and build a customized working graph, enriched with data from third-party plugins. However, Atlassian guarantees the protection of data submitted to Atlassian Intelligence with clear guidelines:
- Data is not used to improve OpenAI models. Atlassian Intelligence only uses data about how you interact with features. For example, the people you work with, the size and type of attachments, and the feedback you provide. Each data request is sent individually to OpenAI, via an encrypted SSL service, to be processed and sent back to Atlassian. OpenAI does not store submitted data or generated responses.
- Atlassian Intelligence can only be used if you activate the option. In this case, there is no fine-tuning of Atlassian Intelligence on your input or output data. There is only training on the feedback you provide. Atlassian Intelligence is covered by Atlassian's terms of use, privacy policy and Atlassian's commitment to the RGPD.
- Administrators have the power to restrict Atlassian Intelligence's access to sites and products of their choice.
Generate and transform content with Atlassian Intelligence
The first set of features, and the most obvious when you think of assistance from artificial intelligence models, is content generation and transformation. Features that can be very useful in Confluence... but not only. Atlassian Intelligence also offers writing assistance for Jira tickets. Here's a demonstration.

Generate content in no time
Confluence
In Confluence, all macros powered by Atlassian Intelligence AI are accessible via the /ai command. The one we're interested in here is the first, which generates text on demand. Using a prompt, i.e. a written request to the AI, simply specify the subject of the paragraph to be written and watch the text scroll before your eyes. You can then modify the prompt completely, or rerun it to obtain several variations of the same command. When you're satisfied with the result, validate the generated text and move on to the rest of the document! It's worth pointing out here that content generation is all the more effective when the prompt is detailed and complete. Don't be afraid to be exhaustive, to include keywords and to iterate through different prompts until you achieve a conclusive result.
Jira Software and Jira Service Management
Jira Software and Jira Service Management also feature automatic ticket content generation. Atlassian Intelligence can assist you in writing your ticket description and comments. Here, AI is not accessible via a command. Instead, you have to click on the Atlassian Intelligence logo, the one that looks like a firework. From there, the logic is the same as on Confluence. And so are the points to watch out for!
There are a few points to bear in mind, however, as content generation, whether in Confluence or Jira, can present a number of limitations. In particular, if :
- You must reference information that is not already present in the document being edited (for example, content present in another document or another product);
- You need to generate and transform content in languages other than English;
- You need to generate content in a format other than standard markdown (for example, generate an information panel from scratch).
On the whole, we still find this feature somewhat limited. Unsurprisingly, it is even more limited in French. The texts generated are frankly not very complete. And don't dare request a text of a certain length, or you'll get a message saying that the prompt doesn't comply with the terms of use. In English, Atlassian Intelligence fares a little better. But the texts generated remain short and do not take into account all the keywords and criteria set out in the prompt. Even after several iterations.
Tips and best practices
Anyone who has ever used ChatGPT will have realized this. The OpenAI model is more than adept at speaking on any subject and, at times, giving the illusion of expertise. The integration of the model with Jira and Confluence is not exempt from these shortcomings. That's why a few tips and best practices are essential. When it comes to content generation by Atlassian Intelligence on Jira and Confluence, bear in mind that :
- Atlassian Intelligence helps you write new content. For example, strategy pages, project overviews, release notes or user stories. But it works best when teams use clear, specific prompts, with a clear objective in mind.
- Break down complex requests into smaller, more manageable tasks. Add relevant keywords to improve the accuracy of generated content. Use correct grammar and punctuation in your input text.
- Experiment with different prompts or variations of your entry text to explore different ideas.
Transforming content
Another possibility in this first series of functions is content transformation. When we speak of transformed content, we're thinking of two cases. The first is a simple reformulation of the content. To give it a more professional or empathetic tone, to correct mistakes or to shorten a text that is too long. All these transformations are available in the Confluence pages, but also in the comments and descriptions of Jira tickets. Simply type /ai to access the various transformation options.
The second is the synthesis or summary of Confluence pages and Jira Service Management tickets. With just one click, you can generate a complete summary of a Confluence page containing a lot of text. Or produce a summary of the elements of a Jira Service Management ticket to quickly understand or recall the context of the ticket. Note that only the comments and description are taken into account for this summary. The elements contained in the fields are not retrieved. What a pity! The Confluence synthesis is already more convincing, even if Atlassian Intelligence's inability to draw on the contents of macros proves annoying.
Tips and best practices
At present, summarizing content in Confluence using Atlassian Intelligence works well for pages rich in text and/or with few macros. In fact, Atlassian reports that this feature works best if the page contains a lot of text. That is, if it takes 5 minutes or more to read it, and there are few visuals and/or other macros such as expand/expand. On the other hand, there are certain situations that make life harder for Atlassian Intelligence:
- If you need precise information about people, places and facts ;
- If you're trying to generate a summary of a very short Confluence page where there isn't enough content. Or a page where the content is mainly contained in macros, tables or expand/expand.
AI-powered search and suggestions
Search for Jira requests in natural language
Atlassian Intelligence offers to translate a request search in Jira into JQL (Jira Query Language), to simplify complex searches. The user accesses the search page in the Ticket tab available at project level in the left side panel. By clicking on the IA icon, you can perform a search using a simple language. Here's an example: I want to retrieve the requests assigned to me that were completed last month. The AI automatically transforms the request into a JQL query. In the example below, even though the spelling is approximate and the query is in French, the result is instantaneous and correct. However, Atlassian points out that queries in English are more efficient.

Beyond language, the limitations remain quite significant. The tool proves interesting when it comes to searching for Jira requests with specific fields and values. However, its usefulness diminishes when it comes to searches involving objects other than requests, such as projects or users. For example, it's impossible to retrieve all the requests a user has worked on in the last 3 weeks. Missing from the result are requests on which the user has been assigned and is no longer assigned, requests where he has added a comment, modified a field, etc.

It is therefore essential to understand the possibilities and limitations of JQL (Jira Query Language). This is to avoid frustration as the AI simply translates what it can, sometimes with disappointing results. In this particular example, the AI has not taken the status into account and fails to correctly translate a date search.

Less SQL in Atlassian Analytics
To take demand research and reporting a step further, there's Atlassian Analytics. The tool is available for Cloud Enterprise plan customers. It enables the creation of data visualizations from Atlassian tools, as well as from external sources.
This tool not only offers report templates, but also the generation of customized views via SQL queries. Atlassian Intelligence now makes such queries possible without the need for SQL knowledge. The main benefit of AI is to compensate for the lack of knowledge of database structures. Natural language queries via AI can be used to better understand and learn the Atlassian Data Lake schema in order to successfully build the desired custom views.

Confluence content search help and suggestions
The Confluence search tool also benefits from the addition of AI. This allows you to obtain answers through quick or advanced searches. These are generated exclusively from the content present in Confluence (page or blog). Please note that if you respect the access restrictions, no content is generated. It is of course recommended to have detailed and up-to-date content in Confluence for best results. If questions are deemed subjective or not detailed enough, they will not trigger a response.

Related sources can be consulted, and users can rate or flag responses.

Simplified automation
You can use Atlassian Intelligence to create automated rules more quickly in Confluence. To do this, a rule must contain at least one trigger and one action. You can also add conditions or branches if you wish to make it more detailed.
Let's take a prompt example: "I want to create a Jira request of type "New feature" in the MobileApp project every time a page in the MOA space changes to the validated state."
AI saves time by choosing the right trigger and the right action.
Even so, there are still a significant number of elements to be configured. The AI's suggestions may have to be repeated (the summary and/or description of the request, for example). Or they may have been forgotten in the request written by the user for the AI. In fact, the automation generation engine is very well designed and intuitive. It guides you step by step through the construction of the automation. However, this is lost by trying to summarize everything in one sentence with the AI.

On the other hand, this feature is a very good approach for a user who is new to automations in Confluence. AI allows you to understand the mechanism by which automations are built, and to quickly familiarize yourself with them.
Future features of Atlassian Intelligence
Extended content summary functionality
For the moment, content summarization is limited to Confluence pages and Jira Service Management tickets. Atlassian plans to extend this functionality to Jira Work Management and Jira Software, as well as to the mobile version of Confluence. Content summarization is also available for documents referred to via Smart links. These can be links to other Atlassian applications or to third-party applications (Google Docs, MS Office or Slack, for example).
Automation generation in Jira
After automations in Confluence, it will be the turn of automations in Jira to benefit from AI. It will surely be possible to make queries such as: "If an Epic is closed while child Stories or sub-tasks are still in progress, then notify those responsible to let them know". These will be transformed into automation instantly and automatically. However, automations require no programming knowledge and already offer templates. This raises the question of the relevance of AI for this use case.
There are a number of limitations concerning automations, and these should be borne in mind when generating them. Obviously, the most important limitation is the number of automations per month, depending on the plan (Free, Standard, Enterprise). So don't use the ease of creation brought about by AI as an excuse to indulge in too much automation. And bear in mind that Jira's performance may depend on it. But perhaps Atlassian's AI will be able to optimize existing automations - dream on!
Code review in Bitbucket
Initially, Atlassian will offer generative AI in the comments editor and the Pull Requests description editor. This will make it possible to summarize or modify the tone of comments before publishing them. The second phase of AI integration in Bitbucket will be to use it to speed up code reviews. This will automatically generate Pull Request descriptions, analyze code to find, check coding conventions, and more. For the moment, there are already 3 plugins (Tabnine, Codeium, Cody by Sourcegraph). They are available on the marketplace and provide these functions.
In conclusion
Atlassian delivers a host of new AI-powered features to further enhance the Jira and Confluence user experience. While some of these features are at best not yet fully developed, or not yet available, we mustn't forget that advances and developments in the world of AI are more than rapid. So don't hesitate to keep a close eye on Atlassian's announcements, and come back to this article to see what features have finally been added to the tools!
Read more articles in our AI (artificial intelligence) series:
What can CIOs expect from AI in 2024?